6 Challenges to Data Integration and How Marketers Can Overcome Them
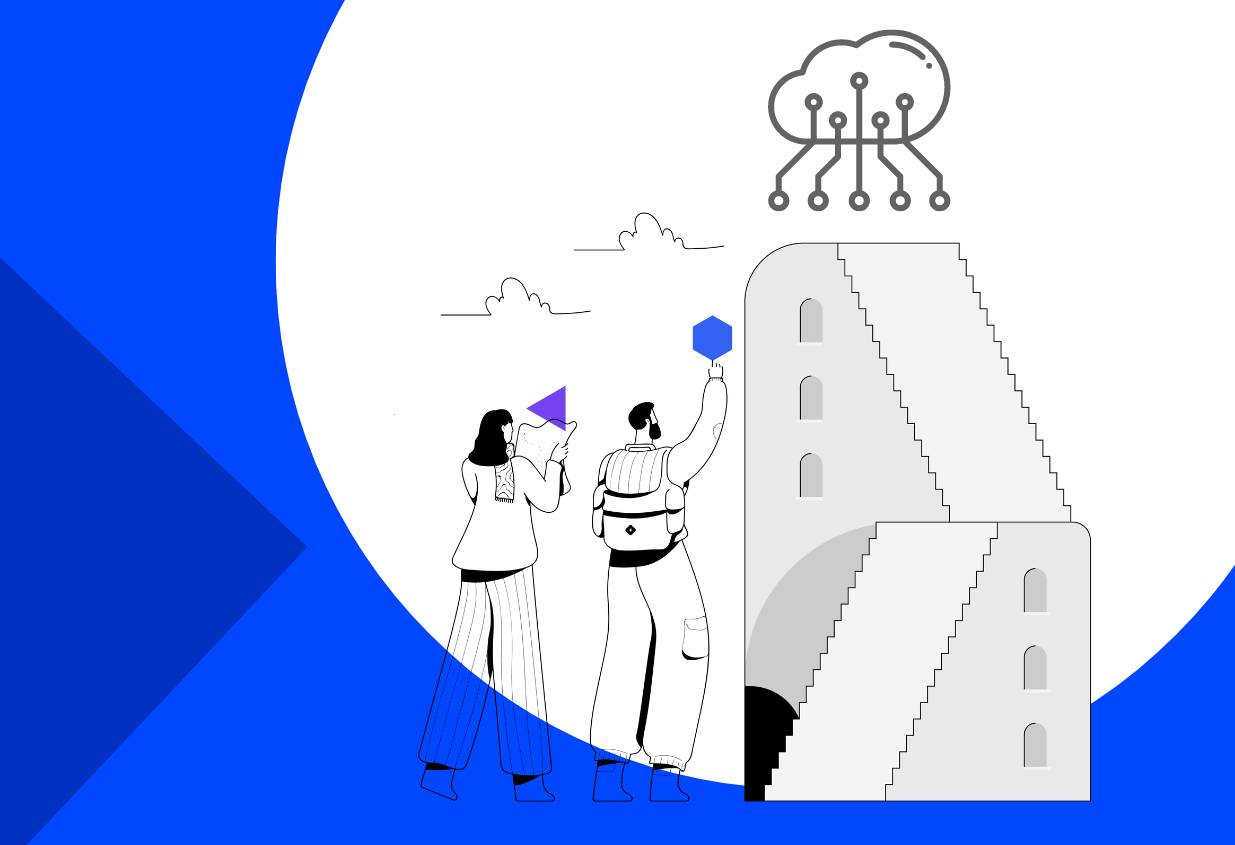
Data integration requires bringing together data from various sources, systems, and platforms to provide insights that can inform advertising campaigns. A robust marketing data integration strategy can transform an agency’s big data into big leaps in productivity, help them scale their business, and prove value to shareholders and clients alike. But what are the hindrances that hold most agencies back from achieving this goal of data integration?
Data integration can be difficult for advertising agencies for several reasons. It can be easy to underestimate the complexity of data integration, which is why we decided to outline common barriers agencies face when seeking to integrate their data. Here we’ll talk about the top six issues we see holding agencies up: data fragmentation, data quality, legacy systems, lack of standardization, resource constraints, privacy and security. After defining each of these challenges, we’ll cover how you can work to move past them to use data integration to propel your agency to the next level.
Data Fragmentation
Advertising agencies often have to deal with a vast amount of data from various sources, including social media platforms, search engines, web analytics tools, and customer relationship management (CRM) systems. This data is often stored in different formats and in different locations, making it challenging to integrate.
The fragmentation has worsened with the addition of AI-generated content, new short-form media platforms, and custom in-house tools that produce even more siloed, often unstructured, data.
Data Quality
Data quality is a significant issue in advertising, as the accuracy and completeness of data can impact the effectiveness of advertising campaigns. Data can be incomplete, inconsistent, or outdated, which can make integration challenging.
Now, real-time performance demands and predictive modeling amplify the impact of poor data quality, making inconsistencies and delays more detrimental to both strategy and automation.
Legacy Systems
Many advertising agencies still rely on legacy systems that are not designed to work with modern data technologies. These systems may not be able to support the integration of data from multiple sources, or they may require manual data entry, which is time-consuming and prone to errors.
In today’s hybrid-cloud environments, legacy systems are also a bottleneck to AI-readiness, lacking the APIs and metadata transparency needed for seamless orchestration.
Lack of Standardization
Advertising agencies often use different tools and systems for collecting and storing data. These tools may use different formats and data structures, which can make it difficult to integrate the data seamlessly.
With the explosion of custom taxonomies and client-specific naming conventions, the lack of a consistent data language across teams, platforms, and campaigns has become even more problematic.
Resource Constraints
Data integration requires a significant investment in terms of time, money, and resources. Advertising agencies may not have the necessary resources, including skilled personnel and technology, to perform data integration effectively. To ensure data integration budgets remain intact, data integration should be culturally positioned within the organization as a journey, not a destination.
As AI Agents and automation expectations rise, many teams now face added pressure to “do more with less,” but still lack the specialized talent to build and manage intelligent data systems.
Privacy and Security
Advertising agencies must also ensure that the data they integrate is compliant with privacy laws and regulations. They must take measures to protect sensitive customer data from theft, cyber-attacks, and other security threats.
Emerging AI regulations and the proliferation of consumer data across intelligent agents have created new layers of compliance risk, especially around data lineage, consent management, and auditability.
Successful data integration requires a strategic approach that addresses these challenges effectively.
How to overcome data integration challenges
Overcoming data integration challenges requires more than stitching systems together — it demands a modern, AI-ready foundation built for flexibility, security, and scale. Agencies looking to stay competitive must embrace a strategic approach grounded in both marketing operations realities and emerging AI requirements. Here’s how forward-thinking agencies are making it happen:
1. Design a Flexible, Future-Proof Data Integration Strategy
Start with a holistic plan that goes beyond just syncing tools. Build a roadmap that prioritizes key data sources, aligns stakeholders around shared KPIs and naming conventions, and includes both structured and unstructured data — including AI-generated content. Incorporate versioning, lineage tracking, and semantic context from day one to support automation and decision-making down the line.
2. Centralize and Normalize All Data — In Real Time
To combat fragmentation, agencies must consolidate marketing data into a unified platform capable of real-time normalization. A cloud-native data lake or warehouse is table stakes — the differentiator now is a system like NinjaCat’s DataCloud that can continuously ingest, transform, and enrich data from hundreds of channels, campaign types, and client-specific formats without manual intervention.
3. Establish a Unified Data Language Across the Org
With every campaign, platform, and client introducing new taxonomies, governance has never been more important. Create a shared semantic layer that standardizes formats, KPIs, and taxonomy structures. This “common language” allows AI agents and analysts alike to work from the same source of truth — reducing friction and increasing velocity.
4. Upgrade Legacy Systems With AI-Oriented Middleware
Rather than ripping and replacing every legacy platform, smart agencies are layering in middleware that connects old systems to modern AI infrastructure. Look for cloud data platforms and tools that support agent orchestration, dynamic ETL, and metadata enrichment to bridge gaps and automate workflows without needing full-scale system migrations.
5. Implement Continuous, Automated Data Quality Management
Data quality isn’t a one-time cleanup — it’s an always-on function. Build pipelines that proactively profile, cleanse, deduplicate, and validate data as it flows in. Integrate anomaly detection, feedback loops, and adaptive logic to catch issues before they derail performance reporting or AI outputs.
6. Invest in AI-Ready Talent & Training
Today’s data team needs more than SQL chops. Getting your data AI-ready means upskilling analysts and engineers in areas like prompt engineering, AI agent orchestration, semantic modeling, and compliance automation. Embed cross-functional roles that connect data ops with client success, ensuring insights drive business outcomes — not just dashboards.
7. Bake Privacy, Security & Compliance Into Your Data Stack
With AI agents accessing more data than ever, agencies must lead with zero-trust architecture and automated compliance monitoring. Ensure your platform supports encryption, role-based access, consent management, and audit trails at every stage of the pipeline — and make sure compliance extends to AI-driven systems, not just human workflows.
Data integration can be challenging for advertising agencies, but it is critical to driving effective advertising campaigns. By creating a comprehensive data integration strategy, consolidating data sources, standardizing data formats, investing in modern data technologies, ensuring data quality, hiring skilled personnel, and complying with privacy laws, advertising agencies can overcome data integration barriers and unlock the value of their data.